

This story initially appeared in The Algorithm, our weekly newsletter on AI. To get stories like this in your inbox initially, register here
I've been try out utilizing AI assistants in my daily work. The most significant barrier to their working is they frequently get things blatantly incorrect. In one case, I utilized an AI transcription platform while speaking with somebody about a handicap, just for the AI summary to firmly insist the discussion had to do with autism. It's an example of AI's “hallucination” issue, where big language designs just make things up.
Just recently we've seen some AI failures on a far larger scale. In the current (amusing) gaffe, Google's Gemini declined to create pictures of white individuals, specifically white guys. Rather, users had the ability to produce pictures of Black popes and female Nazi soldiers. Google had actually been attempting to get the outputs of its design to be less prejudiced, however this backfired, and the tech business quickly discovered itself in the middle of the United States culture wars, with conservative critics and Elon Musk implicating it of having a “woke” predisposition and not representing history properly. Google said sorry and stopped briefly the function.
In another now-famous occurrence, Microsoft's Bing chat informed aNew York City Timespress reporter to leave his partner. And client service chatbots keep getting their business in all sorts of problem. Air Canada was just recently required to provide a consumer a refund in compliance with a policy its consumer service chatbot had actually made up. The list goes on.
Tech business are hurrying AI-powered items to introduce, in spite of comprehensive proof that they are difficult to manage and frequently act in unforeseeable methods. This unusual habits occurs since no one understands precisely how– or why– deep knowing, the basic innovation behind today's AI boom, works. It's one of the most significant puzzles in AI. My associate Will Douglas Heaven simply released a piece where he dives into it.
The greatest secret is how big language designs such as Gemini and OpenAI's GPT-4 can find out to do something they were not taught to do. You can train a language design on mathematics issues in English and after that reveal it French literature, and from that, it can find out to fix mathematics issues in French. These capabilities contradict classical stats, which supply our finest set of descriptions for how predictive designs ought to act, Will composes. Learn more here.
It's simple to error understandings originating from our lack of knowledge for magic. Even the name of the innovation, expert system, is unfortunately deceptive. Language designs appear wise since they produce humanlike prose by anticipating the next word in a sentence. The innovation is not really smart, and calling it that discreetly moves our expectations so we deal with the innovation as more capable than it actually is.
Do not fall under the tech sector's marketing trap by thinking that these designs are omniscient or accurate, or perhaps near all set for the tasks we are anticipating them to do.
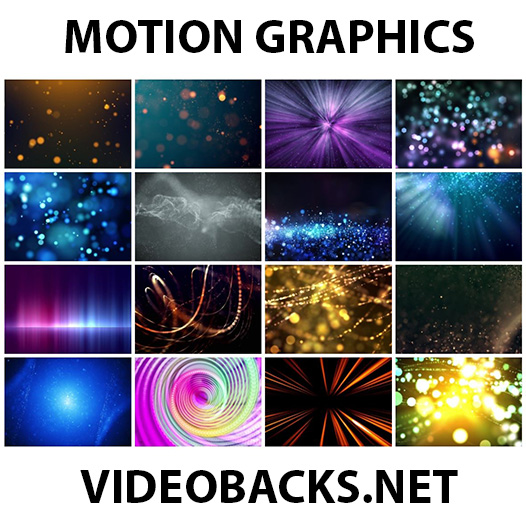