
August 9, 2024 7:28 AM
Credit: VentureBeat made with OpenAI DALL-E 3
Join our day-to-day and weekly newsletters for the current updates and special material on industry-leading AI protection. Find out more
Embodied AI representatives that can communicate with the real world hold enormous capacity for different applications. The shortage of training information stays one of their primary obstacles.
To resolve this difficulty, scientists from Imperial College London and Google DeepMind have actually presented Diffusion Augmented Agents (DAAG), an unique structure that leverages the power of big language designs (LLMs), vision language designs (VLMs) and diffusion designs to improve the discovering performance and transfer knowing abilities of embodied representatives.
Why is information effectiveness essential for embodied representatives?
The remarkable development in LLMs and VLMs over the last few years has actually sustained expect their application to robotics and embodied AI. While LLMs and VLMs can be trained on enormous text and image datasets scraped from the web, embodied AI systems require to discover by engaging with the physical world.
The real life provides numerous obstacles to information collection in embodied AI. Physical environments are much more intricate and unforeseeable than the digital world. Second, robotics and other embodied AI systems depend on physical sensing units and actuators, which can be sluggish, loud, and susceptible to failure.
The scientists think that conquering this obstacle will depend upon making much better usage of the representative’s existing information and experience.
“We assume that embodied representatives can accomplish higher information effectiveness by leveraging previous experience to check out efficiently and move understanding throughout jobs,” the scientists compose.
What is DAAG?
Diffusion Augmented Agent (DAAG), the structure proposed by the Imperial College and DeepMind group, is created to allow representatives to find out jobs more effectively by utilizing previous experiences and producing artificial information.
“We have an interest in allowing representatives to autonomously set and score subgoals, even in the lack of external benefits, and to repurpose their experience from previous jobs to speed up knowing of brand-new jobs,” the scientists compose.
The scientists created DAAG as a long-lasting knowing system, where the representative constantly finds out and adjusts to brand-new jobs.
DAAG operates in the context of a Markov Decision Process (MDP). The representative gets guidelines for a job at the start of each episode. It observes the state of its environment, acts and attempts to reach a state that lines up with the explained job.
It has 2 memory buffers: a task-specific buffer that shops experiences for the present job and an “offline long-lasting buffer” that shops all previous experiences, no matter the jobs they were gathered for or their results.
Diffusion Augmented Agents (DAAG) structure (source: arXiv)
DAAG integrates the strengths of LLMs, VLMs and diffusion designs to produce representatives that can reason about jobs, examine their environment, and repurpose their previous experiences to find out brand-new goals more effectively.
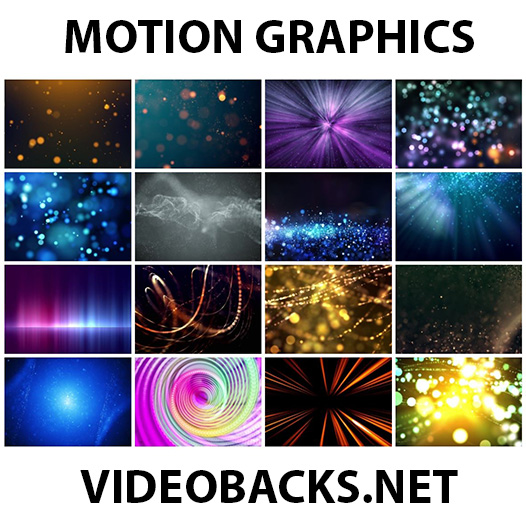