

BitNet is leading the way for a brand-new period of 1-bit Large Language Models (LLMs). In this work, we present a 1-bit LLM variation, specifically BitNet b1.58, in which each and every single criterion (or weight) of the LLM is ternary. It matches the full-precision (i.e., FP16 or BF16) Transformer LLM with the exact same design size and training tokens in regards to both perplexity and end-task efficiency, while being considerably more economical in regards to latency, memory, throughput, and energy intake. More exceptionally, the 1.58-bit LLM specifies a brand-new scaling law and dish for training brand-new generations of LLMs that are both high-performance and cost-efficient. It allows a brand-new calculation paradigm and opens the door for creating particular hardware enhanced for 1-bit LLMs.
The work was done by Microsoft Research and Chinese Academy of Science scientists.
The design not just consumed to 7 times less memory however were likewise as much as 4 times much faster on latency. This enhancement is primarily about memory use being more effective and smaller sized designs in memory size having the very same efficiency or more efficiency. I believe the need for AI abilities and the numerous calculate abilities is pressing for the foreseeable future. I do not believe this will adversely affect Nvidia chip need or its evaluation.
BitNet b1.58 is making it possible for a brand-new scaling law with regard to design efficiency and reasoning expense.
– 13B BitNet b1.58 is more effective, in regards to latency, memory use and energy intake, than 3B FP16 LLM.
– 30B BitNet b1.58 is more effective, in regards to latency, memory use and energy intake, than 7B FP16 LLM.
– 70B BitNet b1.58 is more effective, in regards to latency, memory use and energy intake, than 13B FP16 LLM.
They trained a BitNet b1.58 design with 2T (2 Trillion) tokens following the information dish of StableLM-3B [TBMR]which is the modern open-source 3B design.
New Hardware for 1-bit LLMs
Current work like Groq5 has actually shown appealing outcomes and terrific possible for constructing particular hardware (e.g., LPUs) for LLMs. Going one action even more, we visualize and require actions to develop brand-new hardware and system particularly enhanced for 1-bit LLMs, offered the brand-new calculation paradigm allowed in BitNet.
Brian Wang is a Futurist Thought Leader and a popular Science blog writer with 1 million readers each month. His blog site Nextbigfuture.com is ranked # 1 Science News Blog. It covers numerous disruptive innovation and patterns consisting of Space, Robotics, Artificial Intelligence, Medicine, Anti-aging Biotechnology, and Nanotechnology.
Understood for determining cutting edge innovations, he is presently a Co-Founder of a start-up and fundraising event for high possible early-stage business. He is the Head of Research for Allocations for deep innovation financial investments and an Angel Investor at Space Angels.
A regular speaker at corporations, he has actually been a TEDx speaker, a Singularity University speaker and visitor at many interviews for radio and podcasts.
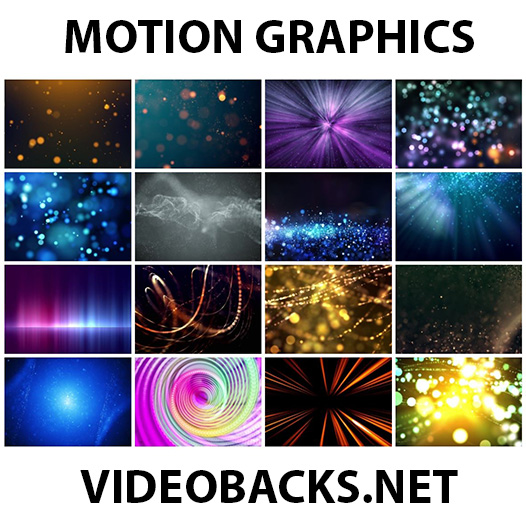