

Visualization of a private information sample as a P-QRS-T wave development seen from 12 leads. Credit: Heart Rhythm (2024 ). DOI: 10.1016 / j. hrthm.2024.07.061
Scientists at Penn State are utilizing artificial intelligence and existing electrocardiogram (ECG) information to assist medical professionals make more precise forecasts. A group of expert system engineers, in partnership with a group of doctors from Penn State Heart and Vascular Institute, is working to establish unique algorithms for point-of-care, internal usage and for innovation licensing.
“With more powerful algorithms and a bigger database, we can forecast cardiovascular results at considerably less expense,” stated Ankit Maheshwari, assistant teacher of medication at Penn State and lead scientist on the task. “And there are a number of other examples out there where it can make healthcare more effective and more efficient.”
An appealing pilot
A preliminary pilot research study released in the journal Heart Rhythm in September reported a design that might forecast whether a client with stroke of unidentified cause would establish atrial fibrillation (AFib)– or irregular heart beat– by examining one heart beat from a reasonably inexpensive and typical heart test, the basic 12-lead ECG, which determines the electrical activity in the heart.
A considerable percentage of strokes of unidentified cause are connected to underlying subclinical, paroxysmal AFib, which is an irregular heart beat that might last for a couple of minutes however the client does not experience any signs.
AFib-related strokes can be avoided by blood slimmers. The existing requirement of care is to implant a loop recorder, a gadget positioned under the skin that tracks heart activity to look for AFib. This assists physicians choose if clients ought to take blood slimmers to avoid future strokes, Maheshwari discussed.
The research study group wished to see if they might utilize the basic 12-lead ECG to forecast AFib rather of counting on a loop recorder. The group assembled a little information set utilizing existing ECG information from Penn State consisting of clients with cryptogenic stroke, or stroke without a clear cause, who had loop recorders implanted, in addition to information from 12-lead ECGs.
Utilizing maker discovering algorithms, the group developed a design that might take a client's 12-lead ECG and forecast whether they would or would not establish AFib. The design properly categorized 80% of clients in the test accomplice.
“This pilot research study reveals that even with a smaller sized group of 200 to 300 clients, we might develop a helpful predictive design,” Maheshwari stated.
Scaling up: Expanding the database, extending the application
Next, the group intends to broaden the database, enabling more comprehensive applications, Maheshwari described. He included that the scientists achieved high levels of precision through information enhancement strategies, which enhanced predictive efficiency.
“Our objective is to arrange the 1.8 million ECGs in the University's medical record system into a searchable database to help with big volume ECG analysis to support future jobs focused on making use of a 12-lead ECG to forecast cardiovascular results and enhance client care,” Maheshwari stated.
Secret to this advancement is cooperation with other organizations,
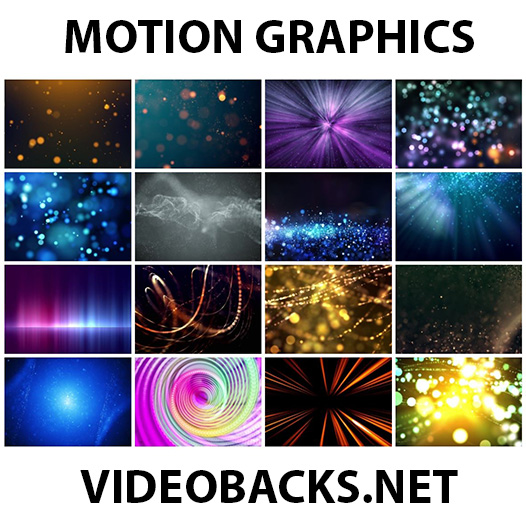