
January 10, 2025 2:05 PM
750″ height=”421″ src=”https://venturebeat.com/wp-content/uploads/2025/01/a-medium-shot-of-a-sophisticated-ai-robo_z7e8_hz3QaqLCZpO3cV2tw_AJOkXZ8wSti6QVF-s9_LZg-transformed.jpeg?w=750″ alt=”VentureBeat/Ideogram”/>
Join our day-to-day and weekly newsletters for the most recent updates and unique material on industry-leading AI protection. Discover more
Hallucinations, or factually unreliable actions, continue to afflict big language designs (LLMs). Designs fail especially when they are provided more complicated jobs and when users are searching for particular and extremely comprehensive reactions.
It's a difficulty information researchers have actually struggled to get rid of, and now, scientists from Google DeepMind state they have actually come an action better to attaining real factuality in structure designs. They have actually presented FACTS Grounding, a criteria that assesses LLMs' capability to produce factually precise actions based upon long-form files. Designs are likewise evaluated on whether their actions are detailed enough to offer beneficial, pertinent responses to triggers.
In addition to the brand-new criteria, the scientists have actually launched a FACTS leaderboard to the Kaggle information science neighborhood.
Since today, Gemini 2.0 Flash topped the leaderboard, with a factuality rating of 83.6%. Others in the leading 9 consist of Google's Gemini 1.0 Flash and Gemini 1.5 Pro; Anthropic's Clade 3.5 Sonnet and Claude 3.5 Haiku; and OpenAI's GPT-4o, 4o-mini, o1-mini and o1-preview. These all ranked above 61.7% in regards to precision.
The scientists state the leaderboard will be actively preserved and constantly upgraded to consist of brand-new designs and their various versions.
“We think that this benchmark fills a space in assessing a larger range of design habits referring to factuality, in contrast to standards that concentrate on narrower usage cases … such as summarization alone,” the scientists compose in a technical paper released today.
Extracting incorrect actions
Making sure accurate precision in LLM reactions is challenging since of modeling (architecture, training and reasoning) and measuring (assessment approaches, information and metrics) aspects. Generally, scientists explain, pre-training concentrates on anticipating the next token provided previous tokens.
“While this goal might teach designs prominent world understanding, it does not straight enhance the design towards the numerous factuality circumstances, rather motivating the design to create normally possible text,” the scientists compose.
To resolve this, the FACTS dataset includes 1,719 examples– 860 public and 859 personal– each needing long-form actions based upon context in supplied files. Each example consists of:
- A system timely (system_instruction) with basic instructions and the order to just address based upon supplied context;
- A job (user_request) that consists of a particular concern to be addressed;
- A long file (context_document) with required info.
To prosper and be identified “precise,” the design needs to process the long-form file and produce a subsequent long-form reaction that is both thorough and completely attributable to the file. Reactions are identified “incorrect” if the design's claims are not straight supported by the file and not extremely pertinent or beneficial.
A user might ask a design to sum up the primary factors why a business's profits reduced in Q3,
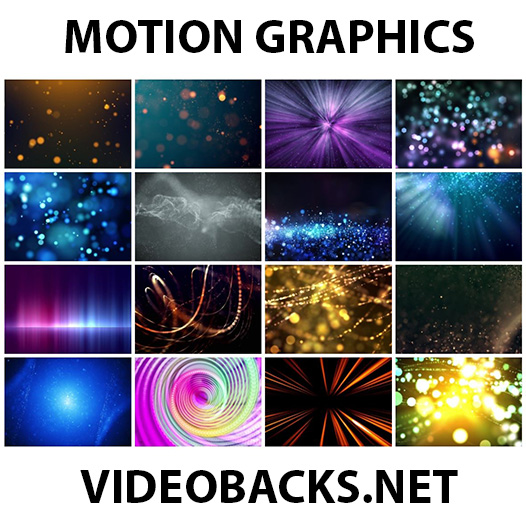