
Back in 2019, a group of computer system researchers carried out a now-famous explore significant repercussions for expert system research study. At the time, device vision algorithms were ending up being efficient in acknowledging a wide variety of items with some recording magnificent lead to the basic tests utilized to examine their capabilities.
There was an issue with the technique behind all these tests. Practically all the algorithms were trained on a database of identified images, referred to as ImageNet. The database included countless images which had actually been thoroughly explained in human-written text to assist the devices find out. This effort was essential for the advancement of device vision and ImageNet ended up being a sort of market requirement.
In this method, the computer system researchers utilized a subset of the images to train algorithms to determine a strawberry, a table, a human face and so on, utilizing identified images in the dataset. They then utilized a various subset of images to check the algorithms. In time, computer system researchers declared that their algorithms were ending up being progressively proficient at acknowledging items in the real life.
Image Recognition
Independently, scientists started to question whether this was actually real. Since the ImageNet database was ending up being so popular, an alternative description was that its images, or ones really like them, were dripping into the real life. AI systems trained on them were simply acknowledging images they had actually currently seen.
At the time, there was no other way to check this due to the fact that there were no premium image databases that had not currently been utilized to train the algorithms.
All that altered when a group from the University of California, Berkeley, produced a brand-new dataset of thoroughly identified images that they understood the algorithms might not have actually seen. They then asked the algorithms to recognize the things in the images and discovered they weren’t as great as everybody had actually declared.
Their experiment ended up being a popular example of the mistakes of depending on single databases for screening makers. Without mindful management of this database, AI systems can appear to be proficient at a job in basic however are actually just duplicating what they have actually currently found out.
That brings us to the existing generation of AI systems which are proficient at fixing specific kinds of mathematics issues drawn up in words. “James composes a 3-page letter to 2 various buddies two times a week. The number of pages does he compose a year?”.
The reality that AI systems can address concerns like this recommends they have the ability to factor. There is an unique database called GSM8K that computer system researchers utilize to check AI system’s thinking capability. This concern is drawn from there.
GSM8K is a “dataset of 8.5 K high quality linguistically varied elementary school mathematics word issues developed by human issue authors.” It includes some 7500 concerns for training an AI system and 1000 concerns to evaluate the system.
Throughout the years,
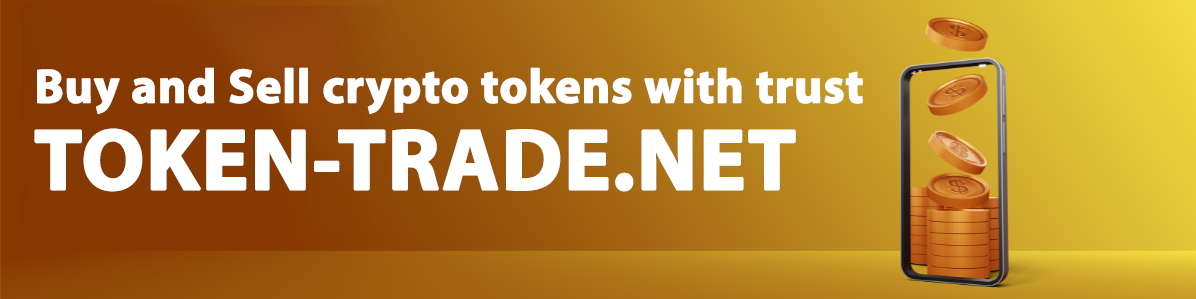