

Bed capability management is of crucial value to health systems, affecting client care and security, functional performance, system sustainability and monetary efficiency. Efforts to enhance and simplify management frequently are separated to areas within the center and might result in suboptimal resource usage, irregular client care, and inadequacies in between care systems for transfers and other care coordination.
Evaluation of end-to-end bed need management internationally from admission to discharge removes much of the unexpected effects of localized optimization efforts. Froedtert Health recognized enhancing capability management as a crucial and targetable objective that might be attained through AI, artificial intelligence and information analytics methods.
Comprehending and dissecting client circulation and its sources enabled the group to produce a suite of predictive tools developed particularly for the care coordination. Froedtert Health had the ability to enhance client care, operationalize essential efficiency indications and enhance operations through more efficient personnel release and usage and by pre-emptively reacting to prepared for modifications in client bed need.
This resulted in enhanced allowance of resources, enhanced client circulation, much better coordination in between departments and expense savings.
Ravi Teja Karri is a maker discovering engineer at Froedtert ThedaCare Health. He and 2 associates will be speaking on these accomplishments at HIMSS25 in a session entitled “Improving Capacity Planning and Bed Demand Forecasting Using Machine Learning.” We talked to Karri to get a preview at what he prepares to go over in March at HIMSS25 throughout his session.
Q. What is the overarching style of your session, and why is it specifically appropriate to health care and health IT today?
A. The overarching style of our session is concentrated on enhancing medical facility capability management and bed need forecasting through the application of expert system and artificial intelligence strategies. This subject is progressively appropriate in health care as health centers deal with unforeseeable modifications in client volume.
Seasonal rises, unexpected admissions and varying client requires make it challenging to preserve an ideal allowance of resources. Leveraging AI and ML to forecast bed need and client circulation makes it possible for health centers to enhance staffing, assign beds and enhance operations, leading to improved client care and general effectiveness.
Our session likewise will check out how health care companies can take advantage of AI and ML to change procedures into anticipatory workflows instead of reactive ones. This proactive method makes it possible for more precise forecasting of client volumes and much better interdepartmental coordination, eventually boosting client experience through more effective resource allowance and prompt care shipment.
Incorporating these predictive designs into everyday operations allows health care companies to much better expect need changes, reduce overcrowding threats and improve interdepartmental coordination.
Q. You are concentrating on AI and ML, crucial innovations in health care today. How are they being utilized in health care in the context of your session's focus and material?
A. Our session concentrates on expert system and artificial intelligence innovations, particularly their application in predictive analytics for bed need forecasting and capability management in health centers. ML designs are developed to evaluate big datasets,
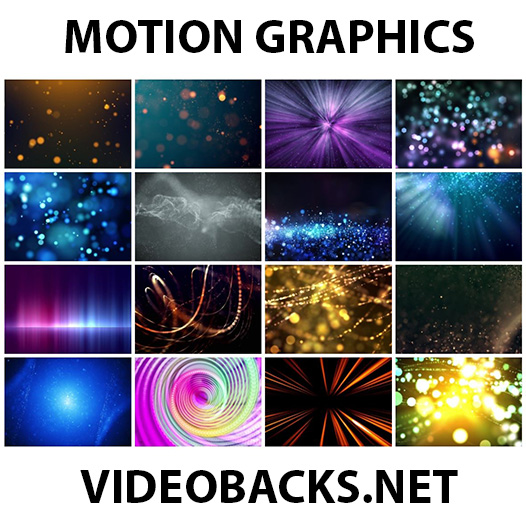